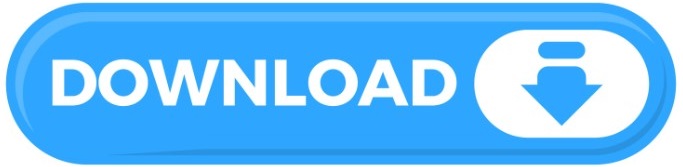
Therefore, this study proposes a hybrid multi-filter wrapper method for feature selection of relevant and irredundant features in software defect prediction. On the contrary, wrapper-based FS have good predictive performance but with high computational cost and lack of generalizability.

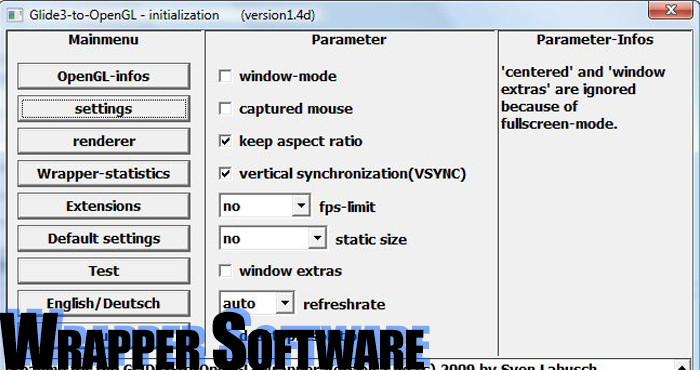
Filter-based FS has low computational cost, but the predictive performance of its classification algorithm on the filtered data cannot be guaranteed. Feature Selection (FS) is a well-known method for solving high dimensionality problem and can be divided into filter-based and wrapper-based methods. The high dimensionality of software metric features has been noted as a data quality problem which negatively affects the predictive performance of SDP models. Nonetheless, the predictive performance of SDP models reckons largely on the quality of dataset utilized for training the predictive models.

It helps software engineer to optimally, allocate limited resources to defective software modules or components in the testing or maintenance phases of software development life cycle (SDLC). Software Defect Prediction (SDP) is an approach used for identifying defect-prone software modules or components.
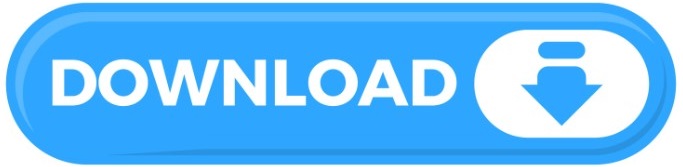